Computex is recognized as the world’s largest tech conference focused on the IT and IoT industry. This year Computex invited Jerry Chen, head of Global Business Development for NVIDIA’s Manufacturing and Industrial Business, to provide an insightful AIoT Forum keynote titled “The Promise of Digital Transformation: How AI-infused Industrial Systems are Rising to Meet the Challenges.”
Most of us already know that AI-driven transformation is impacting every industry in the global economy. What started a decade ago with AI-enabled cloud services pioneered by hyperscale companies has given birth to smart consumer applications, services, and devices.
Today, we are seeing the rise of the next exciting phase of AI for interconnected, complex, industrial-scale systems. This new phase brings the promise of industrial cyber-physical systems infused with AI, with the ability to self-regulate and self-optimize to achieve unprecedented levels of reliability and productivity.
In his address, Chen delved into the AI Journey to look at the waves of AI, digital transformation with industrial AI, the challenges faced, as well as the future of AI. We do know that AI is a force to be reckoned with, and we look forward to seeing how NVIDIA leads the world on the journey to this exciting new phase.
Introducing AI as a General Purpose Technology
Economists call AI a General Purpose Technology, or an innovation that doesn’t serve just one, singular purpose. Like domesticated agriculture, written language, the internal combustion engine, electricity, and information technology, AI broadly impacts all existing industries.
Just as each of these General Purpose Technologies triggered Industrial Revolutions, AI is the spark for the fourth Industrial Revolution. More than that, because AI has the potential to learn, improve, and operate autonomously, it will only get exponentially more powerful over time.
The AI Journey: Waves of AI
The AI journey came in waves, starting with AI Computing which was triggered by the Big Bang of AI when 3 innovations came together a decade ago: machine learning algorithms, lots of data, and GPU computing. The first production deployments of AI started with the big Hyperscalers like Amazon, Baidu, Google, and Microsoft. These Cloud Service Providers were the first to build cloud-based AI services into their platforms.
The next big wave of the AI journey, the AI of Things (AIoT), is represented by AI breaking out of the cloud and extending into industrial assets. This new Industrial Wave presents very different challenges, compared to AI services deployed in managed cloud data centers.
What follows is the Industrial Edge, which is represented largely by remote, unmanaged industrial assets in factories, oilfields, or rail networks. Emerging technologies like 5G are enablers to deploy and manage AI at the Industrial Edge. 5G is also a conduit to collect sensor data to teach Edge AI systems how to continuously learn and improve.
Not all Industrial Edge systems, however, have the luxury of relying on connectivity. Many mission critical applications need Intelligent Agents to perceive their environments and to operate autonomously. Though we may think of these Autonomous Systems as robots, the reality is they are complex cyber-physical systems that continuously learn, adapt, and improve how they operate.
Unlike the First Wave of Cloud-based AI systems, the latter two Waves of AI operate in the wild, outside of managed data centers. Chen emphasized that this AI-Infused Industrial Edge, or AIoT, represents enormous sectors of our global economy.
Growing Industrial Applications of AI
The pandemic, increasingly complex global supply chains, and semiconductor capacity constraints are also collectively driving AI into being a necessity. Its value and impact are felt across a spectrum of industries such as design, manufacturing, supply chain & logistics, data science, and more.
Examples of high-value use cases in industrial applications that we can find today are the deployment of AI for predictive maintenance and service operations and the wide adoption of AI for image-based quality inspection in precision manufacturing.
Chen’s message is clear: every industrial sector will be transformed by AI. Its potential for value creation is enormous, and the spectrum is staggering.
Challenges of Deploying AI for Industrial Applications
To achieve Industrial Digital Transformation, we’ve got to navigate a whole host of unique challenges. Specifically, Chen raised eight unique challenges: connectivity, scalability, manageability, development & prototyping, security, safety, autonomy, and design and optimization.
To combat all these challenges, NVIDIA is investing in solutions to address each of them. Chen cited the example of aluminum beverage can inspection to demonstrate how NVIDIA’s partner Data Monsters deployed AI to inspect 100% of all cans at 60 cans per second. In another innovative example, NVIDIA’s partner V7 Labs developed a platform that enables modern car manufacturers to train inspection systems to recognize defects that are unique to each manufacturing process, and to enable their customers to retrain their AI models using their own unique data. NVIDIA also uses these AI-augmented inspection technologies for its own manufacturing operations.
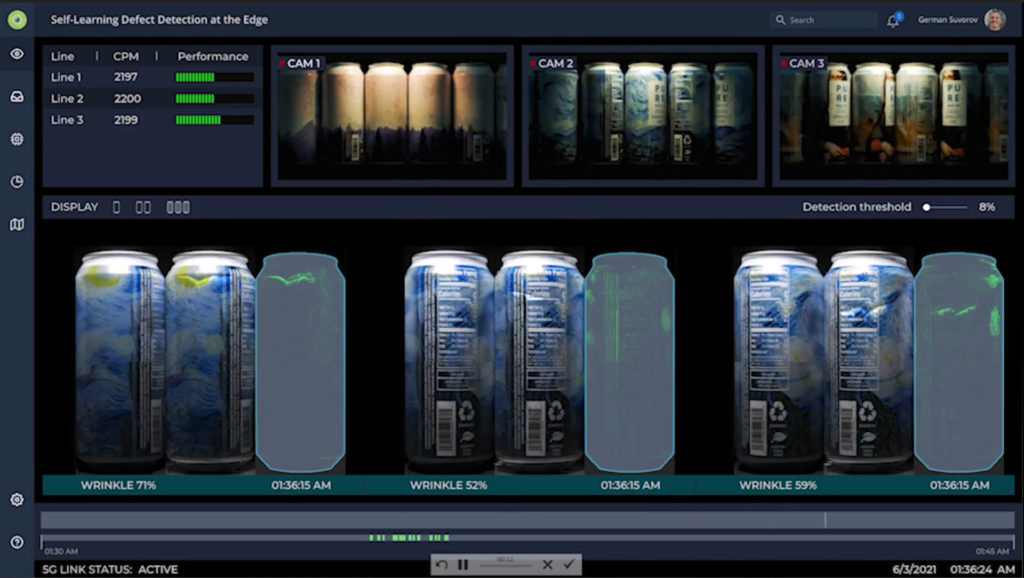
NVIDIA and BMW: Omniverse Platform
NVIDIA and BMW Group’s highly anticipated collaboration came in the form of BMW Group’s Smart Transport Robot and SortBot which uses NVIDIA’s Isaac platform to train their robots in a simulated environment for motion planning, object detection, and pose estimation. The robots are training in a virtual environment using Isaac Sim running on Omniverse to provide a simulated training environment with physical rules and realistic visuals.
This approach, called “sim-to-real”, is designed to train an autonomous AI Agent to safely learn in a synthetic environment. The trained AI Agent is then taken out of the virtual simulation and inserted into a physical robot in the real world. This approach promises to transform how industry will train and deploy robots in all sorts of industrial environments.
Modeling a real, modern, complex manufacturing plant to the level of detail necessary is a daunting task. But BMW Group remarkably achieved this by working with NVIDIA and using NVIDIA’s Omniverse platform, which greatly enhances the precision, speed, and efficiency of this planning process.
Together, BMW and NVIDIA envision a future where a virtual representation of an entire manufacturing production network enables an integrated approach to planning complex manufacturing processes. Like Chen, we look forward to the time when production planners at BMW Group will be able to visualize the entire planning life cycle for every plant in their global production network, accelerated by scalable GPU infrastructure.
Introducing NVIDIA SimNet: AI-Accelerated Simulation Toolkit
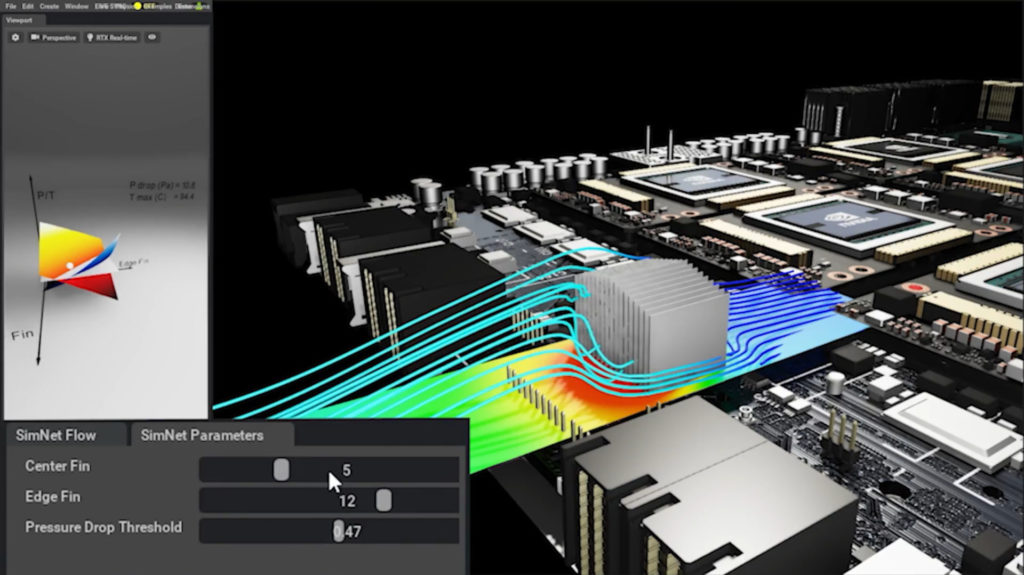
Physics-based models have been used for years to optimize the design and operation of industrial equipment. Now these models are being combined with the true observed behavior of the real physical machines, captured by data from sensors monitoring the physical machines.
Both approaches seek to create digital models of the machines – otherwise known as digital twins. By combining the theoretical physics-based model with the observed reality from data-driven models, digital twins can achieve the best of both worlds using AI.
NVIDIA’s SimNet is a framework for running physics-informed neural networks on GPUs. It illustrates how AI can be infused with an understanding of coupled physics so that it can be used as a surrogate model to explore the design space to find the optimal configuration.
Introducing NVIDIA Morpheus: Cybersecurity as a Strategic Business Imperative
Cybersecurity threats are an issue that everyone is exposed to. But for high-tech companies with valuable IP, this threat is most severe. As modern manufacturing trends towards integration of even more complex and connected machinery, every new manufacturing tool with computing nodes and network connectivity is a potential attack vector. Hence, a cybersecurity solution that is embedded in the very fabric of the communication network is required.
NVIDIA’s Morpheus cybersecurity application framework is therefore a cybersecurity solution designed to enable and work in conjunction with NVIDIA’s DPU network devices embedded in the network fabric.
An open AI application framework that provides cybersecurity developers with a highly optimized AI pipeline and pre-trained AI capabilities, NVIDIA Morpheus allows developers to inspect all IP traffic instantaneously and continuously across their data center fabric. Developers can immediately detect leaked sensitive information as it becomes exposed in network traffic. Bringing a new level of security to data centers, Morpheus provides dynamic protection, real-time telemetry, adaptive policies, and cyber defenses for detecting and containing cybersecurity threats.
NVIDIA’s Expansive Ecosystem Support
We were thrilled to see NVIDIA’s inspiring AI-powered technologies, all of which show NVIDIA’s commitment to creating new markets for its technologies. To make these technologies easily available on the market, NVIDIA’s business model requires relentlessly broad ecosystem support. NVIDIA is available on every major computer platform: on the Cloud, in Datacenters, and in Edge devices. NVIDIA also supports Power, Arm, and of course x86 Intel and x86 AMD platforms.
Ecosystem Building: Moving Forward in the Age of AI
Chen concludes his sharing on an optimistic note by thanking NVIDIA’s ecosystem partners who, along with NVIDIA, are journeying into the Age of AI to transform the future of the Industrial Edge. We are all excited to move forward in the age of AI and journey along with NVIDIA and its partners. Without a doubt, the future of AI—with NVIDIA—is bright.